Academic stories: Chiara Di Francescomarino
ICPM academic storiesNarrated by Chiara Di Francescomarino
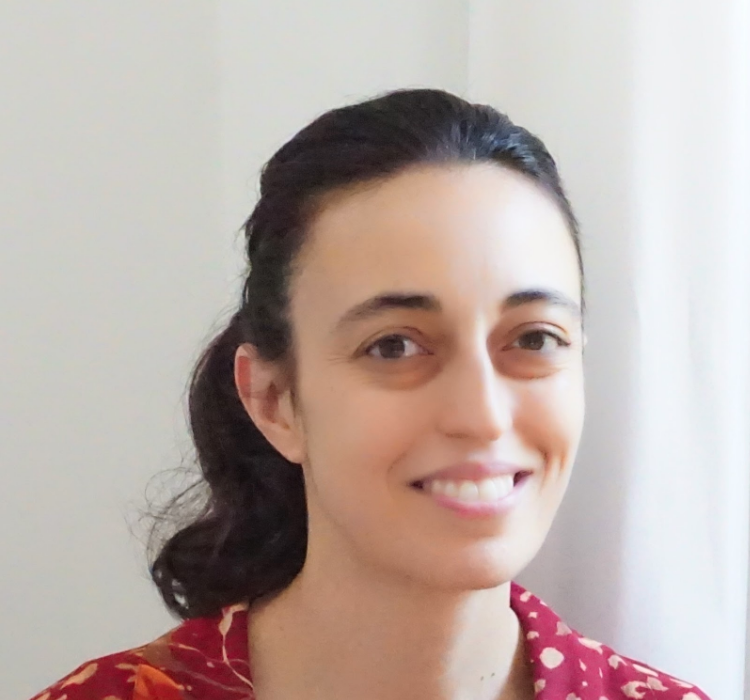
My name is Chiara Di Francescomarino, and I am a research scientist at Fondazione Bruno Kessler (FBK), located in Trento. FBK is one of the most renowned Italian research centres. It is composed of 11 research centres, each focusing on different fields. The ICT area is organised in 6 research centres: Cybersecurity, Digital Health and Wellbeing, Digital Industry, Digital Society, Health Emergencies and Sustainable Energies. I work in the Center for Digital Health & Wellbeing (DHWB). DHWB activities mainly focus on Computer Science and AI as well as on methodologies for health and healthcare. Within the DHWB Center, I work in the Process and Data Intelligence (PDI) research unit, a team composed of three research scientists, two PhD students and many undergraduate students. The activities of PDI revolve around process mining and information extraction as well as their intersection with other areas.
I met the Business Process Management (BPM) community during my PhD studies. Back then, I was working on business process modelling and had the chance to present one of my papers on a process query language. It was a BPM workshop in 2008, in Milan. The first person I met on the first day of the first scientific event I had ever attended was Hajo Reijers. He really made me feel at ease though it was a completely new experience for me. Not to mention that even the community was new to me. Indeed, I carried out my PhD in a software engineering group, which was into software coding, testing, and reverse-engineering – not only from code but also from recorded software executions. While discussing in such a group with my PhD advisor, Paolo Tonella, and other colleagues (Angelo Susi and Alessandro Marchetto), the idea of applying reverse engineering techniques to discover a process model occurred to us as something almost unavoidable.
We applied these techniques for the reverse engineering of a web application. We exercised and tracked the web application GUI, and discovered the process model – a spaghetti-like model, of course. We hence focused on reducing the discovered process model by leveraging multi-objective genetic algorithms in order to optimise multiple objectives: not only reducing the complexity of the model while preserving the conformance between model and traces but also preserving the business information in the reduced model, computed by using domain ontologies. This was my first step in the process mining world, which brought me to discover its fascinating literature starting with process discovery.
After finishing my PhD, I moved to the research group of Chiara Ghidini. The focus of my research shifted from business process modelling to process mining. In 2012-2013, I got involved in a research project with the aim of modelling and monitoring public administration processes, such as the procedure for registering a newborn in the national system. Among the objectives of the project, there were the definition and computation of Key Performance Indicators (KPIs). In this setting, one of the main challenges we had to face was the analysis of execution traces that were not completely tracked by the information systems: some activities were executed manually or tracked by third parties, for instance. To be able to measure the KPIs, we had to reconstruct our swiss-cheesed execution traces first. We did so by taking into account the process model and leveraging the partial information available in the traces. We investigated the problem with multiple approaches and considering different types of information (e.g., control flow alone or control and data-flow together). For instance, we encoded it as a SAT problem and as a planning problem, and we employed different reasoning systems (including planners and model checkers). Starting from that project, I kept on having the opportunity to apply process mining on real data in different domains (e-health, factory ground floor data), which provided inspiration for new research ideas.
During my research visit at the University of Tartu in 2014, I started investigating the predictive monitoring of business processes together with Fabrizio Maggi, Marlon Dumas and Chiara Ghidini. The idea of Predictive Process Monitoring (PPM) is leveraging past historical traces to predict the future of an ongoing trace. Given, for instance, the activities that Alice has carried out so far in the living room, PPM can predict whether Alice will watch TV, at what time she will do it, in case, and what she will be up to right after that. To that end, we started working with classical machine learning techniques (e.g., classifiers and regression models). Also, we investigated different types of pipelines and encodings, using various types of information (e.g., activities only, data payload, or textual information that sometimes could be available together with execution traces). With Arik Senderovich, we started looking into the idea of extracting not only the information from a single trace but also from other concurrent traces for better predictions, as it often happens that there are dependencies between concurrent executions.
PPM has been rapidly growing in the last few years. While several challenges have been swiftly faced in this field, still a lot has to be investigated. Although predictions are becoming more and more accurate, they are still not fully leveraged to support the user beyond the mere prediction. Indeed, users would feel more confident if we could explain to them why those predictions were provided. Also, predictions could be used to recommend to users what to do next or which decisions to make or to provide them with proactive actions to avoid future undesired situations. These are all research directions that I am planning to investigate.
So far, together with Williams Rizzi, we have started exploring how to leverage prediction explanations for improving prediction accuracy. Specifically, we use prediction explanations to identify the features that characterize wrong forecasts and use them to improve the overall accuracy of the predictive model.
Another area of Process Mining I am interested in is the exploration of approaches to combine reasoning based on data with reasoning based on knowledge. We have started investigating this aspect in the field of PPM with Chiara and Fabrizio. Our goal is the improvement of predictions when certain knowledge becomes available. For example, to predict the next activities of a traveller, traditional PPM approaches tend to learn frequent behaviours from historical data. Strikes are not frequent. However, if we know a strike is actually taking place (i.e., certain knowledge is available) logical reasoning could be applied to guide the predictive model towards more accurate outcomes. In our initial analysis with Anton Yeshchenko, we mainly focused on the control flow. Then, we began predicting also the data payload associated with activities.
I am curious to see the challenges that will be tackled by works presented at ICPM 2021. Perhaps some of them will be among the ones I have just spoken about! Last year I had the pleasure to be a co-Chair of the Demonstration track together with Jochen De Weerdt and Jorge Muñoz-Gama. This year, I am glad to be a Program co-Chair of the Research Track together with Claudio Di Ciccio and Pnina Soffer. Although the experience is still ongoing, I can already say that it is exciting. Besides the consolidated areas of the past years, we decided to explicitly have in the call for papers also dedicated themes about process mining fundamental research, including formal foundations, conceptual models and human-centred studies. We are sure that plenty of exciting works about the old and the new thematic areas will be submitted and I expect that my experience will become even more enjoyable and inspiring in the next few months.
If you would like to know more about some of the works I mentioned, please find below a list of useful pointers:
- Works on the reverse engineering of web applications:
- Works on the reconstruction of incomplete traces:
- Works on predictive process monitoring:
- Work on explainable predictive process monitoring:
- This article has been updated on April 8 2021, 21:23.
- Narrated by Chiara Di Francescomarino