Exploring newland: IoT and process mining
exploring newlandTalking with Avi Gal
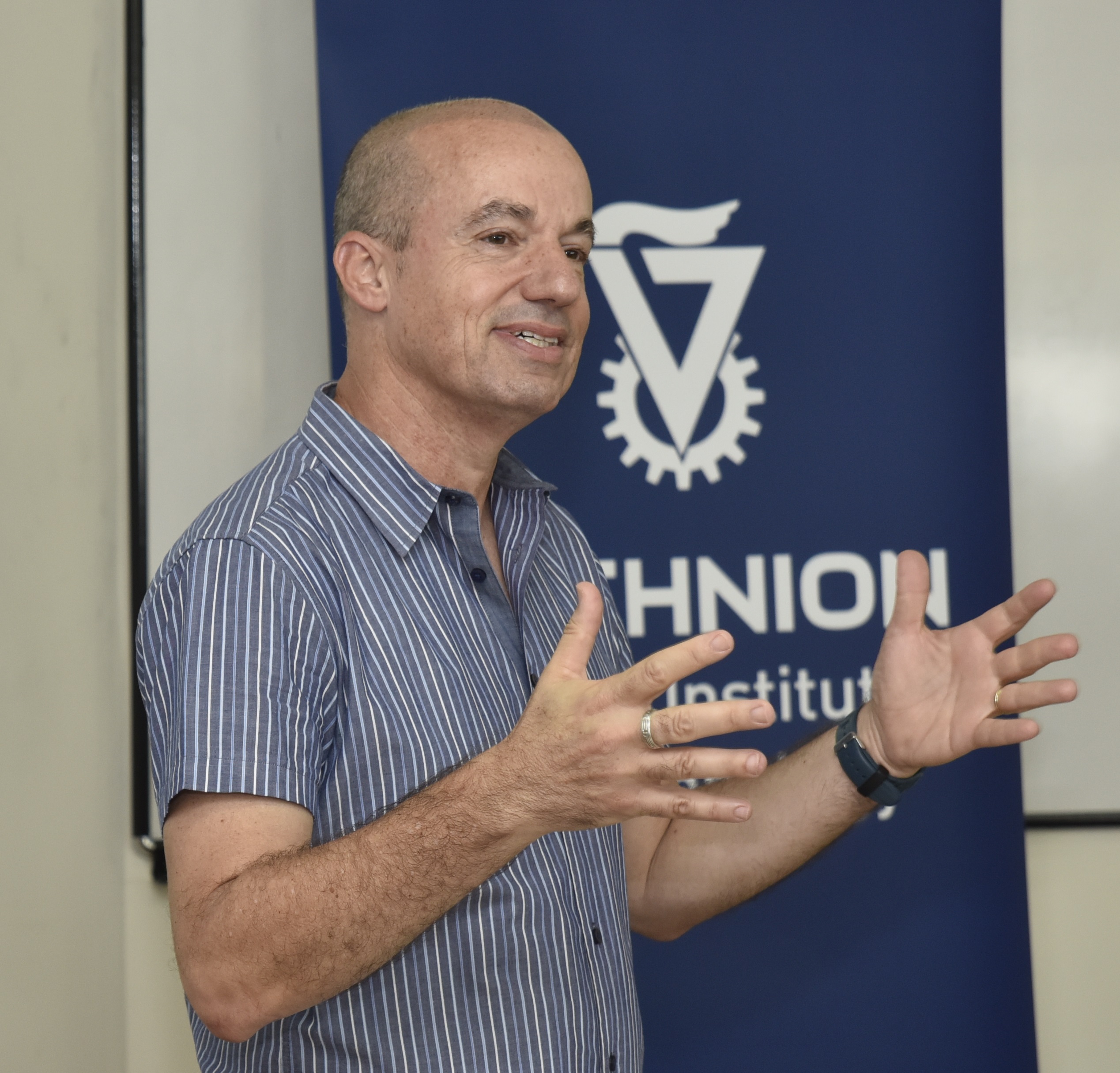
The connection between real-world, interconnected objects and the digital streams tracking their status is becoming the subject of investigation and study for process mining, with new methods and techniques at the crossroads of IoT and process mining. We speak about this new wave of research with one of its most prominent spokespeople, Avigdor Gal.
To start off, Avi, would you tell us a bit about yourself and your research institute?
Hi, my name is Avi (Avigdor) Gal. I am a full professor of data science at the Technion – Israel Institute of Technology. I actually graduated from the Technion many years ago and my PhD work was about connecting two topics that were very trendy in those days, namely active databases and temporal databases. After concluding my PhD I’ve spent two years at the University of Toronto as a postdoc, working in the group of John Mylopoulos and 4 years as an assistant professor at Rutgers University in New Jersey, before returning to the Technion, this time as a professor. The Technion is a leader in data science and artificial intelligence. Ranked number 1 in Europe in csrankings.org, the Technion offers a complete data science ecosystem with undergrad program in data science & engineering, grad program in data science, and a collaborative environment where researchers from all across campus collaborate on data research projects.
When and why did you first come up with the idea to do research in process mining?
As with many other decisions in an academic career, my voyage to process mining happened by sheer coincidence. Arik Senderovich was a Masters student at the Technion and his research was about multi-step shift planning in call centres. During his studies, Arik has realized that the research work he was conducting in service engineering had strong ties to the area of process mining. He brought together his supervisor Prof. Mandelbaum and myself, together with Dr. Matthias Weidlich, then a postdoc in my research group, to conduct an inter-disciplinary discussion on the possible ways of contributing to both research areas. These discussions led to Arik’s enrollment in the PhD program, investigating queue mining, or how to assess system loads from inter-case analysis of logs. His dissertation was recognized with the best dissertation award at BPM 2017.
Why does process mining offer a good toolkit to handle IoT data? And what are the typical 3-5 challenges that darken the nights of a process miner dealing with those data?
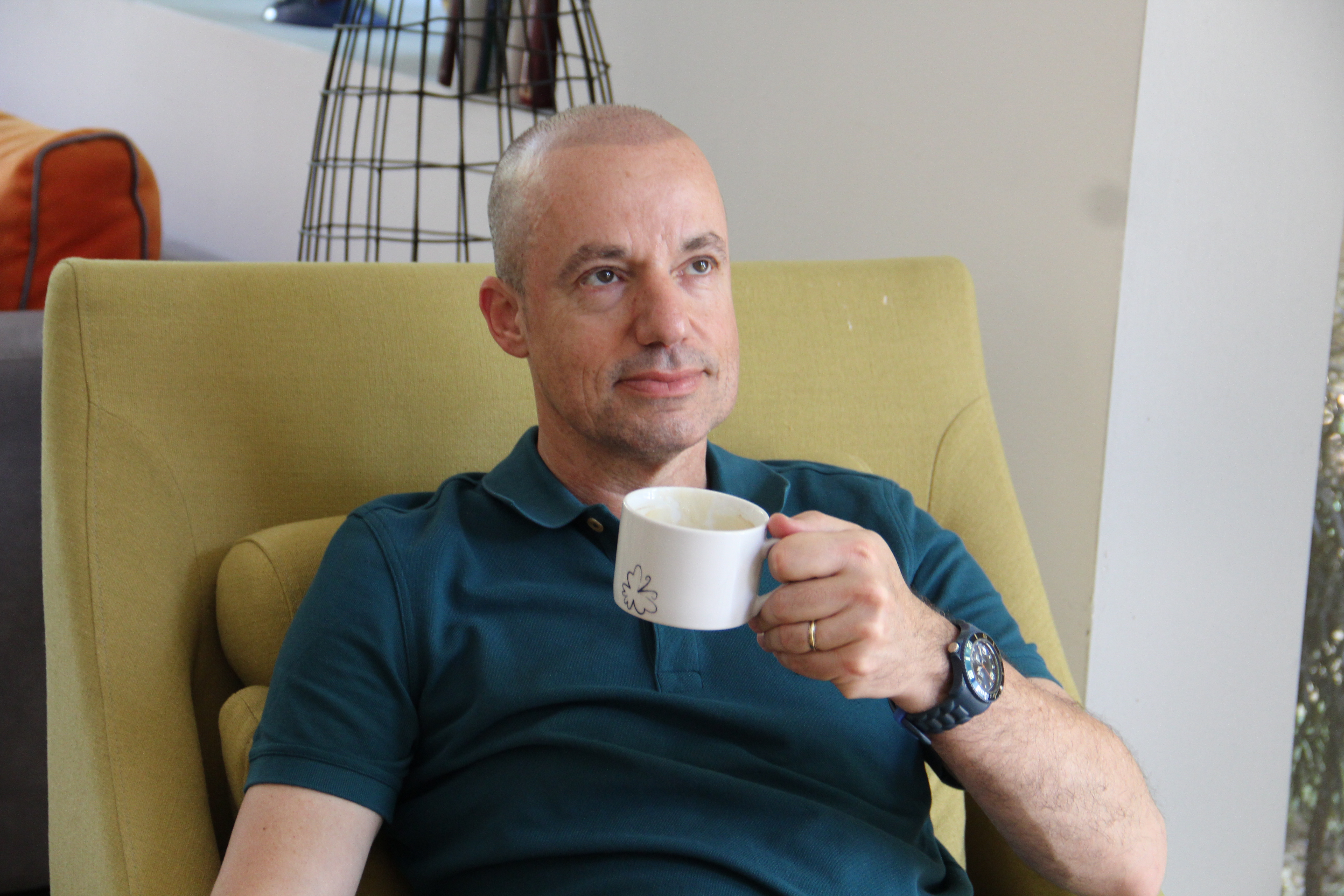
IoT data possess two out of the three main characteristics we seek in process data, namely the who, what, and when. “Who” identifies a case for which the process is recorded, “what” identifies an activity, and “when” timestamps activity occurrence. While IoT data can be typically associated with a sensor (what) and delivers time-stamped data (when), it is oftentimes too low level to offer a “what” that would make sense. For example, consider a smart city application, where buses emit their locations on a regular basis. The bus route (who) and timestamped sensor readings (when) are available. However, the location by itself does not tell enough of the story. Is the bus at a stop? Which stop? is it stuck in a traffic jam? Therefore, there is a need to aggregate IoT readings and annotate them with appropriate activity labels to be correctly utilized for mining processes. Beyond the low-to-high abstraction mapping, there is an issue of data uncertainty that is strongly associated with IoT data. Sensor readings may carry an inherent uncertainty due to sensor limitations (for example, temperature reading, GPS positions, and so on). Also, timestamps may be subject to drifts in local clocks. There may also be a delay in reporting due to network congestion. Therefore, process mining should seek methods to handle uncertainty when discovering new models and when determining conformance.
How do you see the interplay of data science & process mining for real-world objects monitoring in the future?
Process mining has a lot to offer to the data science community in terms of explainable AI. Its origin in the BPM community created research efforts in the directions of data explanation in terms of a process (discovery) and measures to assess distance among models (compliance). The phenomena one can reveal from IoT with data science tools is far from trivial, especially due to the fine-grain availability of data. In that respect, I view the difference between the type of processes that were analyzed when process mining was established and nowadays as the difference between Newton and Einstein. While the former formalized laws that are easily observable by humans, the latter provided insights into phenomena that occur at a too fine-grained level to be noticeable by humans directly. Similarly, with IoT we get readings that are low-level and therefore require “translation” to the application level. This is exactly where process mining fits in, with its explainable tools.
- This article has been updated on December 22 2020, 10:18.
- Talking with Avi Gal