Developers’ point: The bupaR suite
developers pointTalking with Gert Janssenswillen
The bupaR suite is an open-source, integrated set of packages for R, the free software environment for statistical computing and graphics. The bupaR suite helps the user handle and analyse business process data by supporting different stages of a process mining workflow. We speak with Gert Janssenswillen about its inception, core rationale and future development.
Tell us a bit about yourself and your research institute, Gert.
I started working on my PhD in the research group of Business Informatics at Hasselt University in 2014, under the supervision of dr. Benoît Depaire. The previous year, I had a first encounter with process mining during my Master thesis. In a project with the Belgian railway infrastructure provider, we used process mining techniques to measure discrepancies between planned and actual railway capacity usage. This sparked my interest in process mining, academic research, and in many ways paved the way for the creation of bupaR.
My PhD had, foremost, a fundamental nature and focussed on the dimensions of process model quality related to behaviour: fitness, precision, and generalization. Using experiments, I analysed and compared the state-of-the-art quality metrics and their ability to tell us something about the underlying process, which traditionally is considered to be captured in generalisation measures. In my thesis, the inadequacy of existing generalization measures in doing so is shown, and I advocate the use of a two-fold criterium instead of generalization: system fitness and system precision.
I have been doing research in the Business Informatics group at Hasselt University for six years now. Under the lead of dr. Mieke Jans and dr. Benoît Depaire, the research group has developed a strong focus on process mining for quite some time. Within our group we conduct research both on fundamental topics, such as conformance checking and experimental design, as well as on process mining applied in specific business domains, such as audit analytics and healthcare. As a group, we are of course very pleased with the recent appreciation and recognition received by the community. In 2019, my former colleague dr. Toon Jouck already received an honorable mention for the ICPM Process Mining Dissertation Award, for his thesis on the empirical evaluation of process mining algorithms. This year it was my turn, and I am grateful for the recognition that my research received by the BPM Best Dissertation award.
Your PhD also describes and showcases your tool bupaR. What is bupaR about and what is the mission behind it?
bupaR is a collection of R-packages. The name, which stands for “Business Process Analytics with R”, refers to both the overall ecosystem and the core package that makes everything work. The mission behind it can best be described by the four design principles which are used to steer its development: reproducibility, connectivity, extensibility and transparency. Reproducibility ensures that you can easily keep track of your analysis and redo them at a later point in time. Connectivity refers to being able to combine it with other data analysis tools, for instance for machine learning or visualization. Extensibility means that the tool is not only open source, but it is also straightforward to extend with new functionalities. Finally, transparency implies making sure that the tool is properly documented, and its working is both transparent and of high quality.
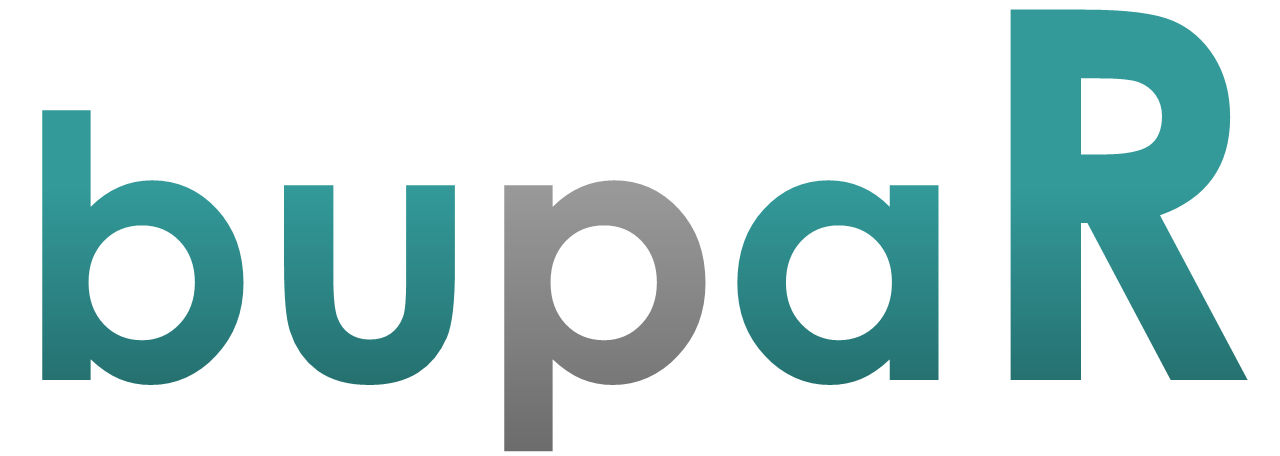
Where did the idea of building a process mining tool with those characteristics come from?
We never started off with a definite goal for the development of a tool in mind. It was the combination of different factors that led to the creation of bupaR. Firstly, during my master thesis project, I realized that there was a lack of readily available process mining tool that allowed you to easily reproduce your analysis at a later point in time. Furthermore there was the need for a tool that was easily extensible with new functionalities and could seamlessly be combined with existing data analysis tools such as clustering or visualization. Of course there was ProM, whose importance for the research community cannot be underestimated and which is extensible with plugins. However, we found it not very straightforward, and ProM somewhat lacks reproducibility and connectivity. While we noticed this void in the process mining tool landscape, the plan to fill it, and in particular by creating a set of R packages, came later on.
Before I started my PhD I already used Python, SQL and other tools such as RapidMiner to analyse data. R had not been in my skill set and it was my supervisor that suggested I learned R as well. At the same time, I was doing a research project together with my colleague dr. Marijke Swennen on the intersection of process mining and lean six sigma. In this project, we developed metrics for descriptive and exploratory analysis of the structuredness and variance of processes. We implemented these metrics in R, and this led to the first published R package for process analysis in 2016. Ever since, fifteen packages have been added to the ecosystem by our group as well as by other researchers.
It has become clear that we were not alone in identifying this gap in the tool landscape. At the time, RapidProm was just introduced, offering a version of ProM that is both reproducible and, to a certain extent, can be combined with existing data analysis techniques. More recently, we have seen the open source tool landscape in process mining extended with PM4Py, which, although providing a different range of functionalities compared to bupaR, is based exactly on the same fundamental design principles.
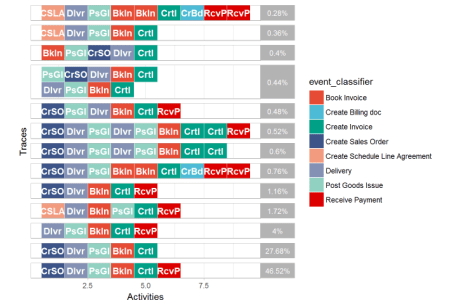
More than anything else, the utility of a tool with these characteristics is shown in the uptake it has received in recent years. Only in the last year, it has been downloaded over 150,000 times in more than 120 countries. For an academic tool, it is remarkable that it is used especially by practitioners, and this in a broad range of fields, such as healthcare, manufacturing, telecommunications, education and business consulting. Case studies and examples have been presented and published by organizations, such as the UK’s National Health Service, BMW, Microsoft, Codecentric, Agenic and Bonitasoft.
While development is still largely done by researchers, dr. Felix Mannhardt (from the Eindhoven University of Technology) and myself leading the effort, we receive a considerable amount of feedback from users that help us to continuously improve and extend the ecosystem.
If you were to characterise your tool in one sentence without superlative adjectives and not mentioning competitors, what would this sentence be?
bupaR provides an easy, accessible and profound toolset, based on scientific research, to analyse business process data, in order to support evidence-based decision making.
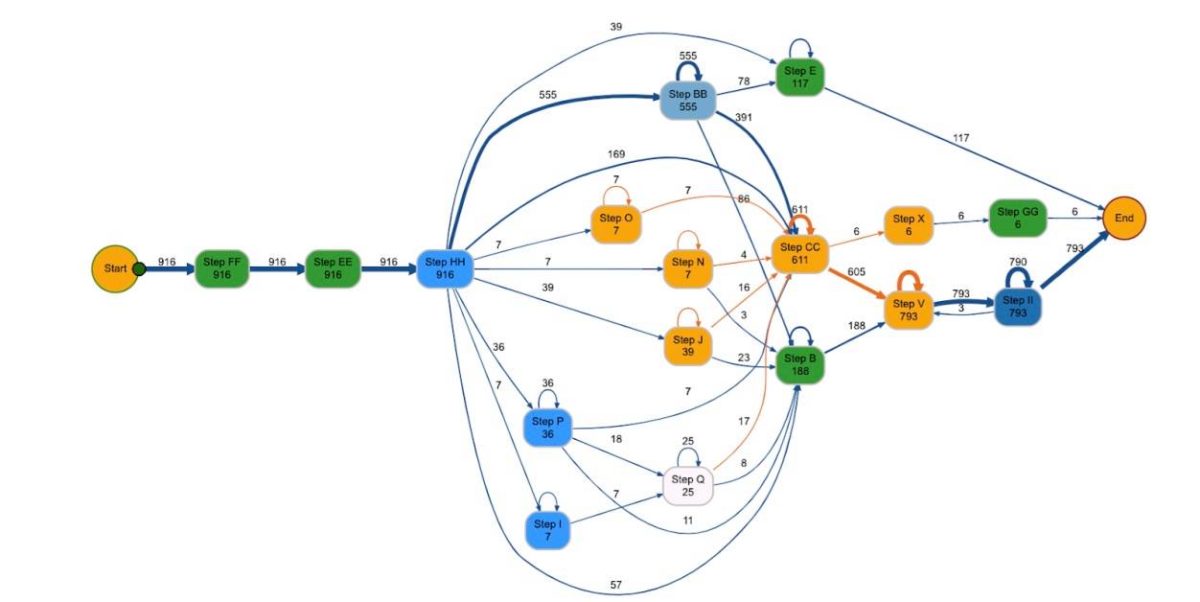
What are your plans for the future of bupaR?
There are some interesting new functionalities which are currently in the pipeline and are expected to become available in the near future, such as implementations of the split miner algorithm and the performance spectrum. We hope to continue adding new features such as those ones to the ecosystem, thereby bringing the users from industry closer to academic developments. In that endeavour, we also want to warmly invite other researchers in the community to consider adding their algorithms to the ecosystem, which will allow them to make their work directly accessible to a large audience of practitioners.
We will also continue working on additional learning materials for users of bupaR, such as new tutorials and cheat sheets. Recently, we published a new online course on Business Process Analytics at bluecourses.com. Part of the revenue that is generated by this course will be used to protect and clean our oceans, so you can help to save nature whilst learning bupaR!
- This article has been updated on December 22 2020, 10:18.
- Talking with Gert Janssenswillen